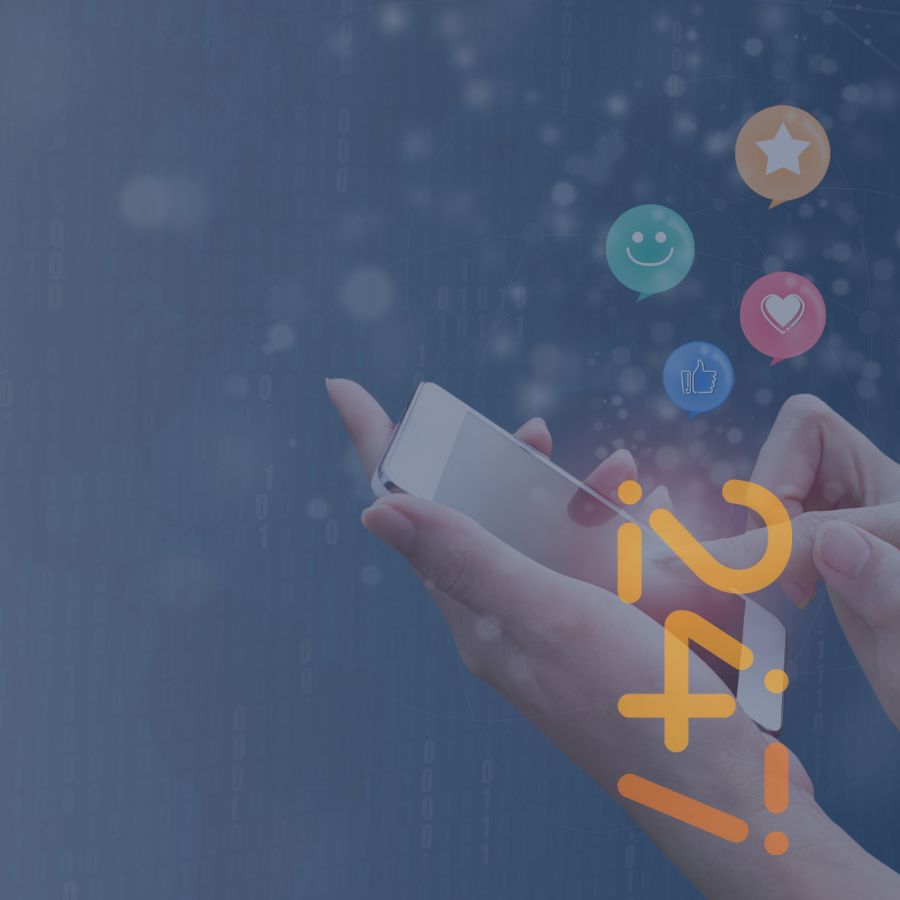
Blog Post
CX measurement is incomplete without unstructured data
Data Fusion - Data Integration - Data Merge
Unlike one of my recent blog posts titled Social Listening - Social Analytics - Social Intelligence, the 3 bigrams in the sub-heading are not part of a continuum, they are synonyms.
Synonyms are words that do not necessarily look or sound alike, but they have more or less the same meaning, while homonyms are words which are spelled the same, although they mean different things.
For the social intelligence discipline, synonyms and homonyms are treated in a diametrically opposite manner: the former are included when gathering online posts whilst the latter must be excluded; failure to do so results in another bigram we so often use in the data analytics business: “Garbage-in…”!
Sometimes, depending on the popularity of the homonyms, more than 80% of the posts gathered - using a social media monitoring tool are irrelevant - referred to as “noise” (as opposed to signal). Only if we have a way to remove the noise can we avoid completing the popular saying mentioned in the previous paragraph with: “…Garbage-out”.
But I digress…
This post is about efficient and meaningful ways to integrate unstructured and eventually structured data sources as part of an organisation's customer experience (CX) measurement or customer management (CM) process - a relatively new more encompassing term gaining ground on CX - in order to discover actionable insights.
This new process of beneficial unstructured data fusion from multiple source types can be described in the following 8 steps:
1. Transform to text
First a quick reminder as to what constitutes unstructured data:
- - Text
- - Audio
- - Images
- - Video
Text analytics is the easiest to perform (as opposed to audio analytics for example) hence the idea to transform all forms of unstructured data to text for easier manipulation.
One of the most useful sources of unstructured data for businesses is their call center audio recordings, with conversations between customers and customer care employees. These audio files can easily be transformed to text (voice-to-text) using specialised language specific machine learning models. An accuracy metric used for the transcripts produced is WER=Word Error Rate which should be lower than 10%.
Another popular source of insights are images e.g. posted on social media or shared on a business client community. A deep learning model adequately customised can produce a caption f describing in text what is illustrated in each image (image-to-text).
When it comes to video, a combination of voice-to-text and image-to-text tech can be used.
2. Ingest on a text analytics platform
When all sources of unstructured data are turned into text, they then need to be uploaded onto a text analytics platform, usually in the form of a JSON or CSV file.
If the same platform has the capability to provide data from additional sources, such as online posts (text and images) from Twitter, Facebook, Instagram, YouTube, reviews, forums, blogs, news etc. so much the better. It can serve as both a social intelligence and text analytics platform.
If needed, text from each source type can be uploaded or gathered and saved separately and merged at a later stage, so as to take a bespoke approach to cleaning and subsequently annotating the text using custom machine learning models for each source.
3. Clean
When it comes to client/user owned data they are all intrinsically clean (read relevant) since the source types are:
- - Email threads between customers and customer care employees
- - Website chat message thread with customers
- - Customer private messages on social media such as Facebook or Instagram
- - Answers to survey open ends
- - Transcripts of qualitative research e.g. focus groups or discussions on online communities
- - Loyalty systems
As for the data gathered from online sources – what is commonly known as social listening or social media monitoring – that is where a thorough data cleaning process is required. The problem as already indicated above is the homonyms. When a Boolean logic query is created to gather posts from social media and other public online sources, using a brand name like Apple or Coke or Orange as a keyword invites a lot of “noise” as you can imagine. The platform is required to offer easy ways to eliminate posts about apple the fruit, cocaine and orange the colour or fruit.
There are two ways to get rid of the irrelevant posts which sometimes make up more than 80% of all posts gathered.
a. Boolean query iterations by adding exclusions for known and newly discovered homonyms after checking a sample of gathered posts
b. Train a custom machine learning model to discern between relevant and irrelevant posts, with the latter treated as noise.
If data cleaning is done properly, we can expect brand/keyword relevance over 90%.
4. Annotate
Natural language processing is the umbrella discipline that takes care of this step in the process. Ideally the use of machine learning models to annotate text in any language works best, but sometimes a rules-based approach may be a shortcut to enhancing the annotation accuracy.
A good text analytics tool offers multiple options i.e. the ability to train generic & custom unsupervised machine learning models or using native language speakers as well as a taxonomy creation feature using a rules based approach.
Text can be annotated for sentiment, topics, relevance, age or other demographics of the author (if not otherwise obtainable), customer journey etc. A minimum accuracy of annotation should be declared and aimed for, and the users need to be able to easily verify the annotation accuracy themselves.
This step can happen before or after the merging of the various data sources, depending on their homogeneity.
5. Merge
For the longest time data fusion or integration or merging from different sources meant weeks or months of data harmonisation, so that the different sources could fit together and make sense. Merging 5-10 source types of unstructured data after steps 1-3 above only takes a few minutes, not months. It would take a few hours from start to fusion.
6. Explore
A powerful filtering tool is required for the user (data analyst) to be able to drill down into the data and discover interesting customer stories which might lead to actionable customer insights. For example, the user could first filter for negative sentiment, then for a specific brand, after that a topic and finally a source type before they start reading individual interactions to get an in-depth understanding of the WHY and the SO WHAT.
7. Deliver
Once the data is cleaned, merged, annotated, and explored, it can be delivered in multiple ways such as:
a. CSV or JSON export of the entire merged dataset with meta data and annotations for each customer interaction.
b. Detailed Excel tables with all possible cross tabs that will enable a market research practitioner or data analyst to produce PowerPoint reports
c. Data in predefined templates for Tableau, Power BI or other platform native or 3rd party data visualisation platforms
d. API access to feed a client’s own dashboards
8. Visualise
Data visualisation via PowerPoint slides, drill down or query dashboards and alerts work best. Ideally the data formats should be flexible so that they can work with multiple data visualisation tools.
Who Is This For?
For now, data fusion included in a CX/CM program is a better fit for larger corporations, for two reasons:
a. They can afford the budget for a continuous 360-degree customer experience measurement.
b. They already have CX measurement and CM programs and dedicated staff in place.
Hopefully soon there will be versions of SaaS products that will make this process efficient and inexpensive enough for SMEs (SMBs) to be able to afford it.
That is what we call the democratisation of data analytics and market research.
Conclusion
The more data sources we integrate the more likely it is for a data analyst, the user of a tool such as listening247, to be able to synthesize actionable insights in their true meaning.
It seems that the biggest gain from this newly found ability to accurately annotate text in any language and fuse/integrate/merge from any source type in a matter of hours is in the discipline of customer experience (CX) measurement and management (CM).
CX and CM are increasingly seeking to encapsulate market research, business intelligence, customer care and other business disciplines and are meant to perfect the customer path to purchase, minimise brand defectors and maximise the number of advocates.