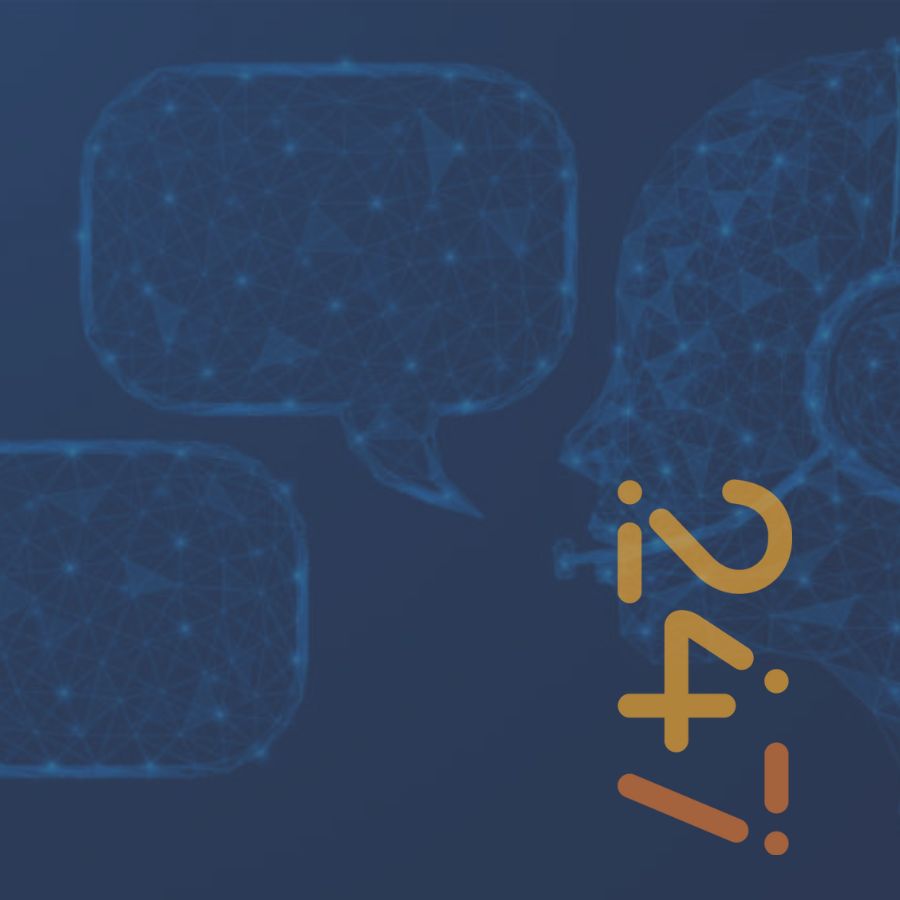
listening247 In the Press
Unlocking the power of CX for business growth with multilingual AI analytics
Text analytics is a transformative tool for businesses, offering the ability to capture and analyze customer opinions across multiple languages. As British executives become more aware of the vast amounts of unstructured data surrounding their businesses, the language-agnostic capabilities of AI in text analytics emerge as a critical, yet often overlooked, component. Unstructured data, which makes up the majority of available information, provides invaluable insights into customer experiences. Leveraging AI for multilingual text analytics enables companies to access and understand customer feedback from diverse sources, ensuring a comprehensive view of customer sentiment and priorities.
The power of natural language processing
The basis for AI-powered text analytics is a combination of machine learning (ML) and natural language processing (NLP).
ML is the way to produce AI designed to mimic human learning. While conventional programming requires the implementation of rules created by humans, ML uses data analysis to learn hugely complex patterns that can be used to infer – making ML powerfully adept at solving problems and performing complex tasks.
NLP, meanwhile, pertains to processing language – in fact, it can be understood as one of the complex tasks that ML supports.
The uses for NLP in this context are many and varied. It can be used for simpler goals, like working out how often a given term or word appears in a text. Alternatively, it can take on the tougher challenge of determining the sentiment – or even emotion – of a given piece of text.
Obviously, both the former and the latter have great utility for businesses who want a detailed understanding of all available customer opinion.
These uses of NLP allow companies to assess enormous quantities of data to discover how often their brand is being talked about online or offline – and whether it’s being perceived positively, negatively, or in relation to a range of more nuanced sentiments.
Multilingual methods
Crucially, as mentioned above, the power of this approach rests in its capacity to encompass all customer opinion – text analytics work with every opinion, rather than a sample or selection.
In order to realise this goal, however, you can’t limit the language in which a given opinion is expressed – you need your AI to be entirely language agnostic, especially if you are a multinational organisation.
We achieve this by using both unsupervised and supervised ML. Supervised ML means that the algorithms involved are ‘trained’ by human beings who annotate training data, allowing the AI to do a much better job than humans when it comes to narrow tasks involving large quantities of data – also known as Big Data.
To ensure that all languages are catered for, we make use of a network of some 300 native speakers of various languages who read, understand, and manually annotate unstructured data – establishing, for example, whether a given Tweet is positive or negative, its topic, the presence of sarcasm, or even the customer journey stage implied by the content of an email or chat message thread.
Once an AI has been trained in the native language (without translating into English and using an ML model for English) to very accurately achieve its goals – whether to establish sentiment or identify the presence of a topic – the results can be easily visualised in English, unlocking the accumulated opinions of all customers for CX professionals, retention managers, and so on in a language they can understand.
On top of this, AI precision can continuously increase. Precision can be measured when a small sample of tweets, for example, are annotated by a human with a certain sentiment. We’re seeing 80-90 per cent or more match the algorithm, irrespective of the language in which the tweets were written.
Bearing in mind the subjective nature of expressing sentiment, this demonstrates just how formidable these AI techniques have become.
Finding needles in an unstructured data haystack
I began this piece by pointing out that UD is everywhere, and that it represents an opportunity to get a sense of all customer opinion – as opposed to polls and surveys which, by definition, can only provide customer opinion based on a sample.
In order to truly achieve this unlimited degree of access into consumer opinion, however, multinational companies don’t just need to engage AI experts and their tech for the English language – they also need to make sure their AI is trained on data across all pertinent languages with the same high precision as for English.
In so doing, text analytics become not only source agnostic, but language agnostic too – allowing business leaders to confidently assert that their understanding of their customers’ views, pain points, and gain points is detailed, precise, and unprecedentedly comprehensive.
Conclusion
In conclusion, to fully leverage the power of text analytics, businesses must recognize the importance of language-agnostic AI. By embracing multilingual AI capabilities, companies can accurately capture and analyze customer opinions across all languages, ensuring a comprehensive understanding of customer sentiment. This approach not only enhances customer experience insights but also provides a competitive edge in an increasingly globalized market. As AI technology continues to advance, the ability to process unstructured data in any language will become essential for businesses aiming to stay ahead.
Origal Source: Global Banking & Finance Review