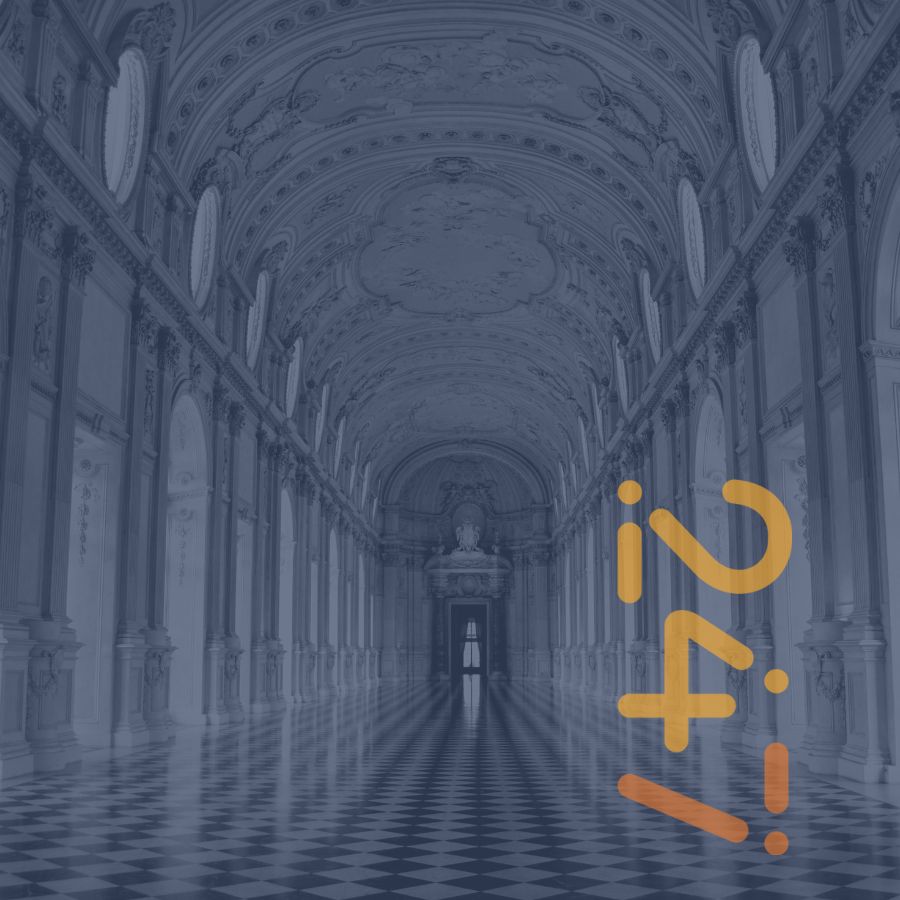
listening247 In the Press
Public sentiment data, the Queen and Boris – Why research into your favourite Royal and not so favourite politician has big business implications!
listening247 recently published a report revealing that, over the last quarter, the Countess of Wessex, Princess Anne, and Camilla were the most positively talked about Royals in the UK, while (unsurprisingly to some) Prime Minister Boris Johnson was one of the UK’s most unpopular politicians – and his cabinet was in the doghouse as well! How do you interpret the results and what insights do they give us into the mindset of the British public?
First of all, it’s worth quickly noting the main KPIs used to rank the subjects of this research:
1. Share of Voice (SoV) – which is calculated based on the total volume of posts for each subject. The total number of posts within a category was used as the base to calculate the shares.
2. Net Sentiment Score (NSS) a metric coined and trademarked by listening247, simply calculated as follows: share of positive sentiment minus share of negative sentiment.
Under many circumstances, a high SoV is great news – but not for Boris Johnson, whose Net Sentiment Score (NSS) rests at -5%. This means, as the graph below indicates, that more online posts about Johnson were driven by negative sentiment than by positive, giving a strong indication of the public’s mood towards their Prime Minister.
Fig 1. NSS Politicians Dec-Mar
Johnson isn’t alone in his negative NSS status. In fact, of all current Cabinet members, only Steve Barclay has a positive score – albeit with a low volume of only 22,000 posts when compared with Johnson’s princely 10 million.
As for the rest, the numbers speak for themselves. Sajid Javid continues to have the worst NSS, with -26% up from -34% during the previous 3-month period. Rishi Sunak sunk from one of only 4 politicians with positive NSS during the last period to the 2nd from bottom with -19% NSS. Priti Patel improved her position from second to last and -24% to an impressive 21 percentage points increase to -3% (still negative, though).
What kind of conclusions might we draw from these results?
For one thing, this analysis of sentiment might give an indication of who may (or may not) be in the running for any prospective Tory leadership battles. Liz Truss is often named as a possible leadership hopeful, for example, but our analysis shows that Truss is perceived twice as negatively as Johnson, suggesting that she’s not currently an optimal candidate.
Setting our sights a little higher, it’s also worth noting that this data was gathered before the local elections – and I think it’s no coincidence that this largely negative perception of the leading Tories was swiftly followed by a loss of around 400 council seats.
In other words: analysis that involves every data source has a great deal of predictive potential.
How about the Royals? What’s the sentiment story there?
In terms of SoV, Prince Andrew overtook Meghan Markle and the Queen who had the highest SoV during the last period – mainly, no doubt, due to the court settlement. Megan is now 3rd with the Queen having surpassed her by 10 percentage points. Now the world makes more sense!
Sophie (+59%) Anne (+56%), and Camilla (+47%) continue to have a higher NSS than the Queen; added to the list of royals with better NSS than the Monarch are Kate, Meghan, Charles, Harry and William in this order. These results might challenge our assumptions that the Queen is the be-all and end-all of Royal discussion – in fact, the Queen’s NSS dropped from 55% to 27% in this period.
Fig 2. NSS Royals Dec-Mar
Given that DMR utilises the latter, is it possible to put on your layman’s hat and explain the process as to how you acquired and interpreted the data for your report?
Unlike traditional polls and surveys, which rely on small sample sizes and can be prone to inaccuracies, listening247's report uses social intelligence technology to gather and analyze unstructured data from a wide range of online sources. By collecting posts from platforms like Twitter, Facebook, blogs, and forums, as well as internal data from clients, the analysis captures unsolicited, honest opinions from the public. This comprehensive approach offers a more accurate reflection of public sentiment, free from the biases and limitations of conventional polling methods.
How far has sentiment analysis come over the last decade and how important is it for customer experience management (CXM)?
Sentiment analysis only sprang into existence at the beginning of the last decade.
From a place of obscurity twelve years ago, however, its rise has been meteoric. Sentiment analysis has truly jumped into the light in this decade – and it’s unquestionably here to stay.
The process is best known for its application in a social media context, but it can be equally useful when applied to other customer feedback sources such as call centres, private messages, emails, answers to open ended questions in surveys, and many more sources besides.
In fact, any source of unstructured data can be considered grist to the mill of sentiment analysis – especially when it’s backed by robust artificial intelligence (AI).
With this AI capability, CXM professionals working for brands can now know with certainty what each and every customer interaction with their brand means and represents.
Why is it only now that we are starting to use unstructured data and what potential does it bring society?
Frankly, because we haven’t had the technology.
Today, however, machine learning and AI has become more mainstream and accessible. Without AI, the only way to understand unstructured data was to read, listen to, or view every single piece of data – which is an impossible task, of course, when you reflect on the millions of posts, calls, chats, and other data needed to gain a truly universal and comprehensive view of customer opinion.
Should people be concerned about their online conversations being analysed?
In a word: no.
For one thing, UK personal data protection laws are fairly robust – as anybody who undertakes GDPR compliance training can attest.
Besides, the strength of our analyses is that the opinions we find and work with are volunteered by their posters. This doesn’t just have good implications for the reliability of the data – it also means that this is data that people don’t mind being publicly available.
Of course, we should all take responsibility and think carefully about what we post in public online spaces – but this is just good general advice which would apply irrespective of any advances in social intelligence technology.
You work with some of the world’s biggest brands such as Bloomberg, Nielsen and Procter & Gamble. Are you able to give us some examples of your insights and project work for them?
There are many use cases whereby machine learning and AI is applied in order to decipher big unstructured data. When it comes to companies like Bloomberg, they see an application for social intelligence as a means of factoring alternative data into trading and investment decisions for their hedge fund clients. Agencies, on the other hand, use social intelligence in order to discover insights based on unsolicited customer opinion for the brands of their big corporate clients. More broadly, of course, literally all companies want to optimise their customer experience by discovering and fixing customer pain points early and efficiently by listening in to all the customer interaction in calls and chats.
How do you see sentiment analysis impacting government and business in the next couple of decades?
In the next couple of decades there are at least two areas where we will see wonders:
- Reactive solutions to new problems: businesses and governments alike will be able to identify problems and pain points for their customers and citizens and resolve them in a matter of milliseconds.
- Proactive solutions: the predictive capability of AI will provide actionable foresight in order to avoid tangling with a predicted problem before it materialises.
Conclusion
Tracking net sentiment scores for public figures, like politicians and royals, on a frequent basis is crucial. As technology continues to evolve, traditional polls may become obsolete, replaced by more accurate and comprehensive methods of gauging public sentiment. For businesses, tools like the listening247's social presence score offer valuable insights, enabling brands to benchmark against competitors and identify the most impactful elements of their campaigns. This approach ensures a clearer understanding of what resonates with the audience and what falls short.
Origal Source: Global Banking & Finance Review